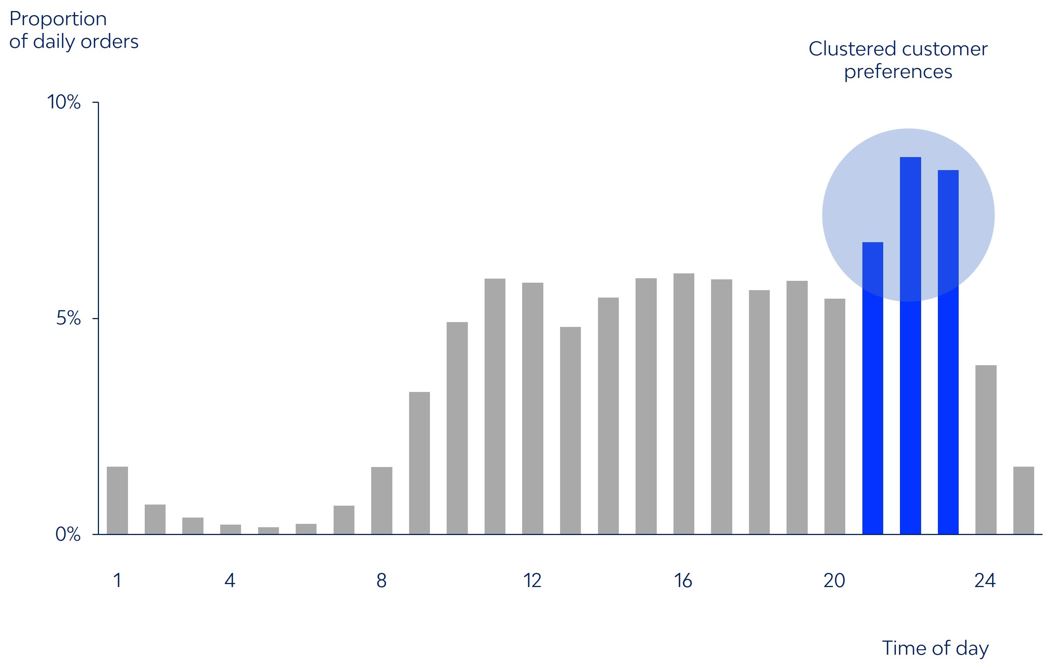
Motivation
Time Slot Selection in e-commerce is the proof of concept of TRUST’s effectiveness in a
prescriptive setting within the retail sector, bringing together INESC TEC, LTPlabs, and SONAE
MC.
Sonae MC is the largest Portuguese food retailer and has an e-commerce operation that, similarly
to other retailers, has been boosted by the COVID pandemic. It is, thus, of the utmost
importance to achieve operational efficiency while not disregarding customer satisfaction.
The current operational inefficiencies in the e-commerce operation come mainly from customers'
preferences, which are clustered around the same delivery window, causing high operational
loads. These operational peaks require an oversized operation and result in a service level hit.
The misaligned customer preferences and supply chain objectives call for a solution compromise.
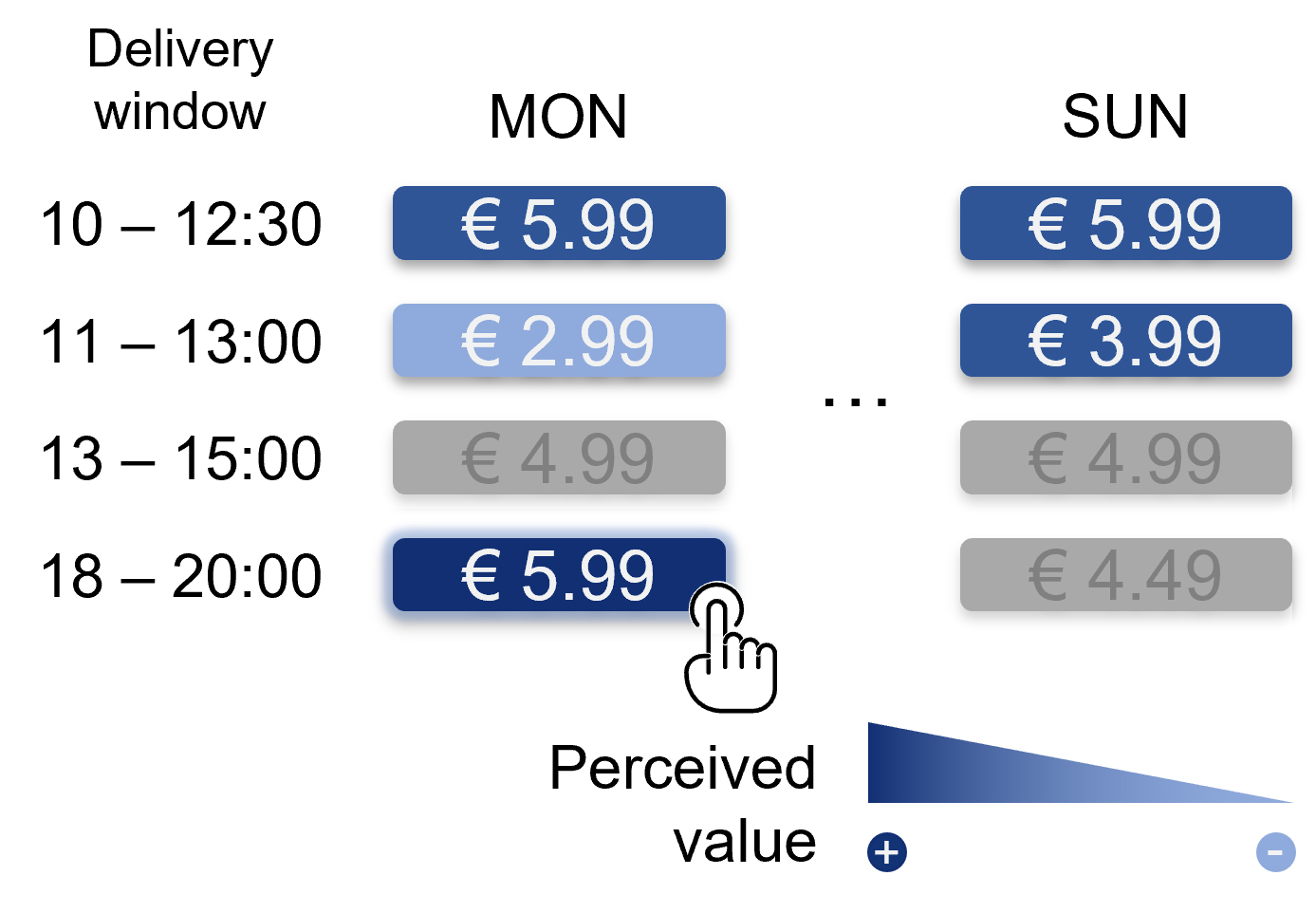
Challenge
Time slot management includes defining the available slots (time windows) that customers may
pick for the delivery of their order and the pricing of each slot. Time slot selection may
pursue a static approach, where slots and pricing decisions are defined beforehand and do not
change during the operation; or dynamically, where the decisions are adjusted based on real-time
orders, taking into consideration the current operational load of each time slot and the
geographic proximity of the customers. Pricing decisions can also take advantage of customers’
preferences.
The objective of this case study is to apply TRUST to dynamically select delivery time slots and
corresponding pricing for last-mile delivery, trading-off customer satisfaction and operational
efficiency. Providing explanations to the prescriptive engine’s output is key in ensuring
customers are handled fairly and decision-makers adopt its recommendations.
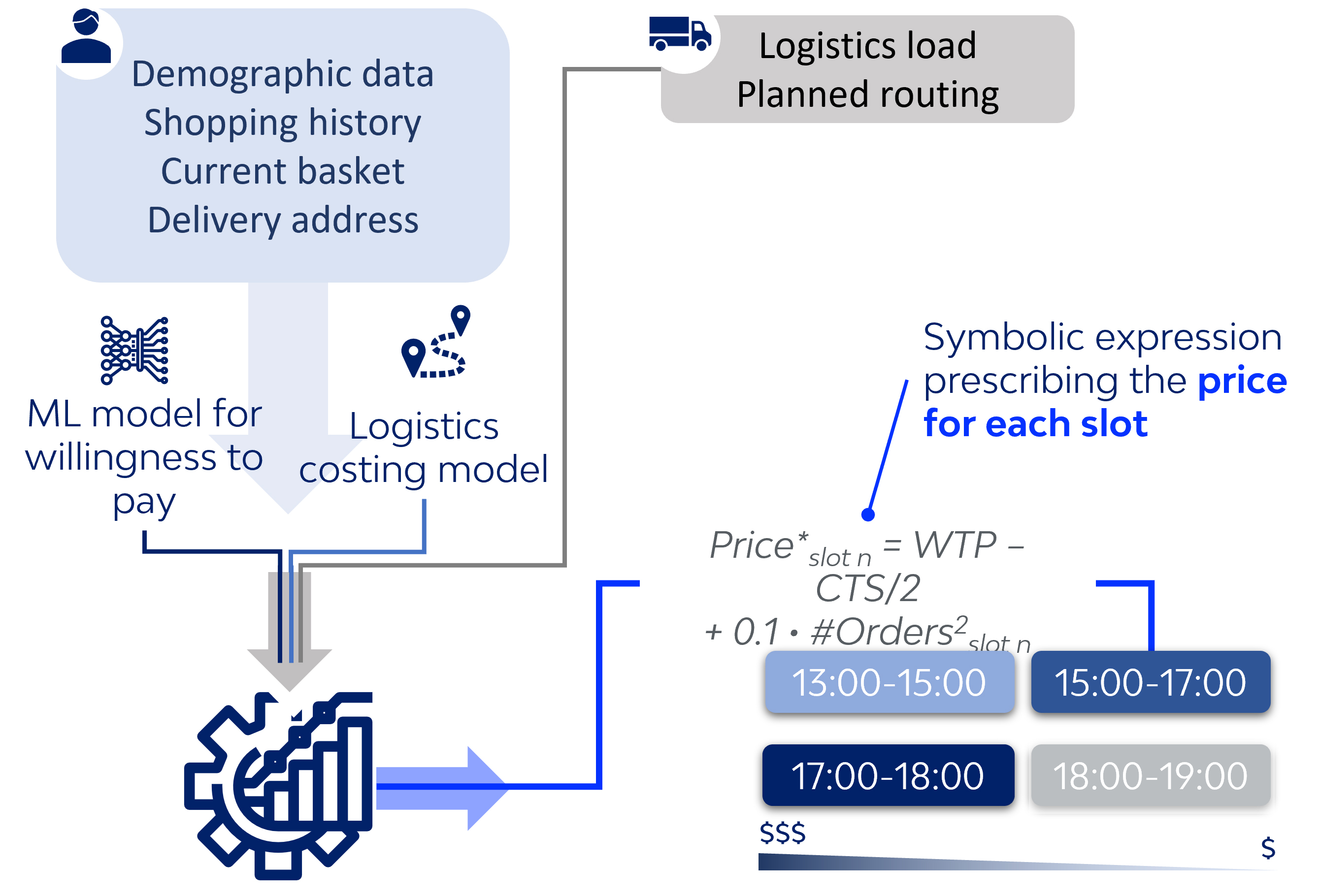
Approach
In this project, time slot management will be targeted using symbolic expressions that will yield the explainability and speed that these operational decisions require:
- At first, customer and logistics data will be leveraged to feed two models: a machine learning model that will forecast customers’ willingness to pay for each slot – pricing pair, and a logistic costing model that will calculate the overall distribution cost as a function of the logistic variables.
- Then, a prescriptive engine will trade-off customer’s preferences and logistic load balance and return a symbolic expression that defines time slots and pricing, while allowing to understand the obtained recommendations.
This symbolic expression, combined with experts' knowledge, increases the comprehension of the dynamic pricing problem. TRUST will enable two types of interactions: At the solution level, by easing cognition while interpreting a particular solution, and at the model level, by trading-off different factors such as performance, explainability, and fairness and favor one or another, depending on the moments.