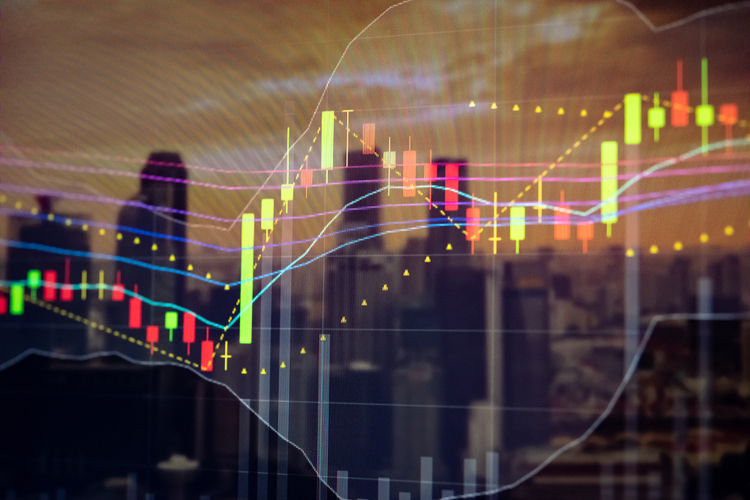
Motivation
Demand forecasting is the scientific backbone of several practical, business and policy uses cases in the energy sector. Forecasting may span over various time horizons, serving different, every time, mandates. In TRUST AI forecasting is addressed in a two fold way. First, at the building level and the short term, driven by real time data, where it can be the basis for several decisions, related to behaviour, system performance as well demand response schemes and dynamic pricing. And second at the country level and the mid term, driven by yearly data, where it supports key policy decisions for CO2 reduction and the evolution of the energy system.
Additionally, the ambition is to deliver and demonstrate a XAI enabled platform where energy real time data and energy applications may be shared under well defined policies. This, sharing, idea we consider as a practical approach and a sound condition for the gradual emergence of an ecosystem in Energy XAI. The short and mid term forecasting applications described briefly above will provide a first illustration of all this, in a practical and tangible way.
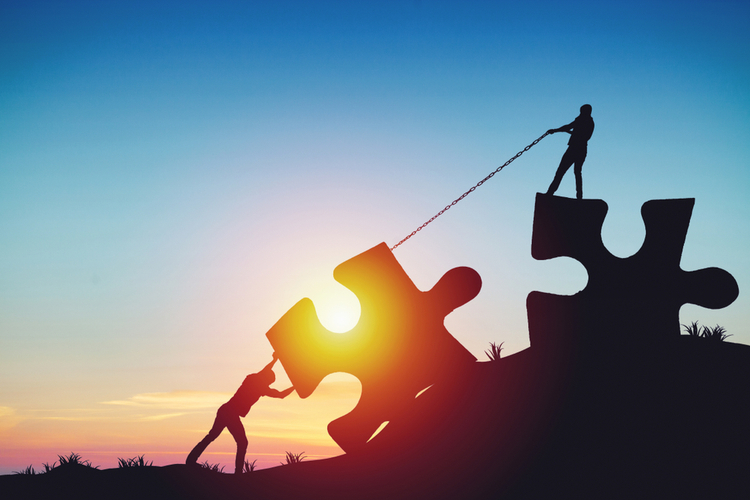
Challenge
Meeting the goals of energy transition beyond the carbon era will require significant advancements in material, process and data management technologies, as well as a better understanding and shaping of user behaviour into more eco-friendly profiles. For example, smart grids and in particular demand response schemes, hold a significant potential for the optimisation of the energy system, which is, however, currently lagging behind in deployment. A main reason for this is the important risk users are feeling there are exposed to. Tools to provide confidence and trust in the potential impact and benefits of the adoption of new pricing schemes can greatly add in the proliferation of such greatly beneficial schemes.
In TRUST-AI Energy we look forward to providing state of the art solutions, insight and advice in the two time horizons addressed. Trust and explainability will in both be key value drivers.
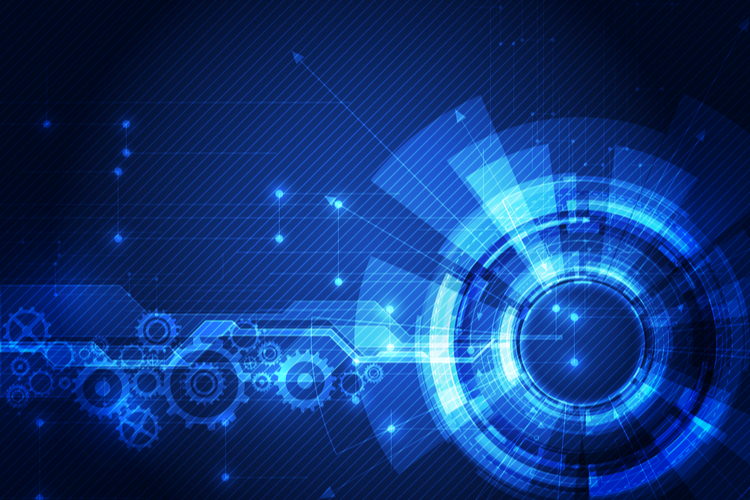
Approach
Real time, high resolution data management is the foundation of the data sourcing, for the building level use case. Neural networks can provided for high prediction accuracies but need to be complemented by insight, trust and an understanding of their results. To this extent, both counterfactual driven local explainability as well as global explainability via diverse surrogate models will be experimented upon in both sub cases.
Additionally, in line with the aim to set the foundation for an ecosystem based on sharing data and apps, TRUST AI in Energy will seek to integrate the TRUST AI framework in its environment and make it available to third parties for experimentation, under policies that will be sorted out within the consortium. Indeed, creating public value for a EU funded project is a key aspect of our broader approach that we will attend to meticulously.